Unsupported: Post-vaccination adverse event reports alone aren’t proof that vaccines caused the adverse event. Simply because an event occurred after vaccination doesn’t mean that the vaccine caused the event. While temporality, or the occurrence of one event after another, is essential for drawing a causal association between two events, it is insufficient on its own to demonstrate causality.
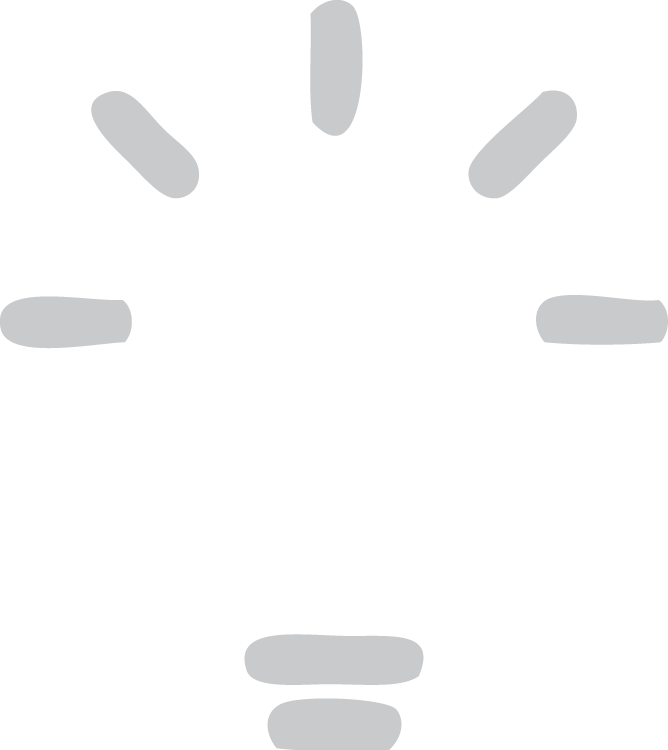
AFFIRMATION COMPLETE: Currently, we see 16 serious side effects per 100,000 vaccinations, and the number of fatal side effects is at 4.11/100,000 vaccinations. For three deaths prevented by vaccination we have to accept two inflicted by vaccination.”
REVIEW
A study published in the journal Vaccines in late June 2021 claimed that there were two deaths caused by COVID-19 vaccines for every three lives saved by the vaccines, and concluded that COVID-19 vaccination showed a “lack of clear benefit”. The study was picked up and shared on multiple social media platforms including Facebook, Instagram, Twitter, and Reddit (see examples here, here, and here). On Facebook, it received more than 31,000 interactions according to social media analytics tool CrowdTangle. Among viral Facebook posts related to the study was a video by political commentator Liz Wheeler, which drew more than 255,000 views to date.
The authors of the study aimed “to determine the effectiveness of the vaccines and to compare them with the costs in terms of side effects”. To determine vaccine effectiveness, they used a metric called “number needed to vaccinate” (NNTV)—which the authors calculated using data from a study conducted on more than 1 million healthcare workers in Israel[1]—with the number of post-COVID-19 vaccine adverse events reports submitted to the Netherlands Pharmacovigilance Centre Lareb.
None of the authors have expertise in epidemiology, virology, or vaccines. The lead author Harald Walach is a psychologist and a faculty member at the Poznan University of the Medical Sciences. His co-authors are Rainer Klement, a medical physicist at the Leopoldina Hospital in Germany, and Wouter Akema, whose affiliation in the paper is described as “independent data and pattern scientist”.
The publication of this study prompted two scientists to resign from the journal’s editorial board: Florian Krammer, a professor at Mount Sinai Icahn School of Medicine, and Katie Ewers, an immunologist and associate professor at Oxford University. Ewers described the publication of the study as “grossly negligent”.
Hi John, I was made aware of the paper yesterday, read it, sent an email to the editorial staff with exactly your question and then resigned from my role at the journal.
— Florian Krammer (@florian_krammer) June 26, 2021
I have resigned from the Editorial Board of @Vaccines_MDPI following the publication of this article. It is grossly negligent and I can't believe it passed peer-review. I hope it will be retracted. @MDPIOpenAccess @ratripp #AcademicTwitter https://t.co/wIFLJanZQP
— 🕷️The wife scientific🕷️ (@ProfKatieEwer) June 27, 2021
Following an Expression of Concern by the journal on 28 June 2021 regarding the study’s “misinterpretation of the data and the conclusions”, the study was ultimately retracted on 2 July 2021. The journal’s editorial board explained that the decision was taken to retract as “the article contained several errors that fundamentally affect the interpretation of the findings” and that “The authors were asked to respond to the claims, but were not able to do so satisfactorily”.
This review explains why the methodology used by the authors is flawed and misleading, ultimately giving readers the false impression that the vaccines’ risks are equal to or greater than its benefits.
Post-vaccine adverse event reports alone aren’t evidence of a causal association—but when combined with other information can show causality
To calculate the number of deaths caused by COVID-19 vaccination, Walach et al. used post-vaccine adverse event reports in the Dutch pharmacovigilance database Lareb. This system is similar to the U.S. Vaccine Adverse Events Reporting System.
As has been pointed out on several occasions—and on the Lareb website (see translation in English)—post-vaccination adverse event reports alone don’t constitute evidence that the vaccine was the cause. However, the manner in which these reports were used by Walach et al., such as their labeling of adverse event reports of death as “deaths by vaccination”, demonstrates that the authors attributed causality to the vaccines based solely on these reports.
In the authors’ response to the retraction of their study, reported by Retraction Watch, they defended the manner in which they used the database, stating that although “the self-reporting system of side-effects for vaccines and other drugs is far from foolproof, it is the only data we have. So why should it not be put to use?”
This line of questioning appears to imply that adverse event reports aren’t used to study potential causal associations, when in fact public health agencies are using such reports to do so. This is evident from the associations observed between blood clots and viral vector vaccines like the AstraZeneca-Oxford vaccine, as well as heart inflammation and RNA vaccines like the Pfizer-BioNTech vaccine. These associations have been widely reported in the media (see here, here, here, and here).
What sets these investigations by public health agencies apart from that of Walach et al. is that they also involved comparisons with baseline incidences of such events. Examples can be seen in these reports here and here by the U.S. Centers for Disease Control and Prevention.
This is an important step, because even before COVID-19 vaccines were available, cases of blood clots, heart inflammation, and death already occurred at a certain rate in the population. In order to assess the plausibility of a causal association between an event and a vaccine, one must first determine if the incidence of an event is higher in vaccinated people than in unvaccinated people.
Walach et al. didn’t conduct such comparisons and failed to show that death occurred at a higher frequency in vaccinated people. Furthermore, previous investigations by health authorities showed that deaths aren’t more frequent among vaccinated people as compared to unvaccinated people.
The metric “number needed to vaccinate” doesn’t reflect vaccine effectiveness
The authors measured vaccine effectiveness by calculating the “number needed to vaccinate” (NNTV, sometimes abbreviated to NNV). NNTV originates from an epidemiological metric known as “number needed to treat” (NNT). In simple terms, NNT is the number of people that need to be treated in order to avoid a specific bad outcome, like death: “if a drug has an NNT of 5, it means you have to treat 5 people with the drug to prevent one additional bad outcome”.
In the same vein, NNTV is the number of people that need to be vaccinated in order to avoid a bad outcome (e.g. death). We can calculate NNTV by dividing 1 with the absolute risk reduction (ARR).
ARR is calculated by subtracting the rate of the bad outcome in the treated group from that of the control (untreated) group. For example, if the rate of the bad outcome in an untreated group is 10%, but the rate in a treated group is 5%, then the ARR is five (10 – 5 = 5).
The authors’ use of NNTV, a value derived from ARR, as a measure of vaccine effectiveness is incorrect. This is because ARR doesn’t account for indirect benefits of vaccines and is influenced by factors like disease prevalence, as explained in an earlier Health Feedback review, which can change independently of vaccine effectiveness. Vaccinologist and associate professor at the University of Auckland Helen Petousis-Harris pointed out in a blog post that “Vaccine effectiveness is never calculated by using an NNT/NNV. It cannot account for the effect on transmission and herd effects of vaccines”.
Since vaccines help a population to approach herd immunity, the more people in a population are vaccinated, the fewer opportunities a disease has to spread. This directly impacts disease prevalence in a population—the higher the number of vaccinated people, the higher the NNTV. That is, more people need to be vaccinated to prevent a bad outcome. The high NNTV isn’t because the vaccine is ineffective, but because the probability of someone getting the disease is now lower due to herd immunity, which in turn makes the number of a specific bad outcome even smaller.
Another factor that influences NNTV is time. The NNTV calculation by Walach et al. was made using data from a study conducted in Israel which lasted for just six weeks[1]. Therefore, the NNTV calculated by Walach et al. only reflects the level of benefit over six weeks rather than over the long run.
But since vaccines can provide benefits in the long term, using data derived from a short-term study to infer a vaccine’s benefits “can make a vaccine seem less promising than the effect that a vaccine would have on a person’s risk over a longer period of time”, as explained by health experts at Meedan Health Desk.
Epidemiologist Gideon Meyerowitz-Katz highlighted the same issue on Twitter.
8/n This means that the NNTV from this study is INHERENTLY MISLEADING unless you assume that vaccines will stop working entirely after the 6-week period (obviously false)
— Health Nerd (@GidMK) June 27, 2021
And finally, the prevalence of an infectious disease can be reduced by measures taken to mitigate the spread of the disease, such as physical distancing and mask-wearing, as pointed out by health experts at Meedan Health Desk. When this happens, the ARR calculated for a vaccine can be small. This isn’t because a vaccine is ineffective. Rather, it is because the number of cases occurring in a community is small.
Conversely, when the spread of a disease is high, the number of cases that occur—as well as the bad outcome associated with that disease—also rises. This leads to a higher ARR. This shows how ARR can change not because the vaccine’s intrinsic effectiveness changes, but as a result of differences in epidemiological situations.
These factors illustrate how NNTV can differ independently of vaccine effectiveness, making its use as such by Walach et al. incorrect.
Conclusion
In the end, the study by Walach et al. failed to address the aim stated in the introduction of their study, which is “to determine the effectiveness of the vaccines and to compare them with the costs in terms of side effects”.
The use of adverse event reports as evidence of vaccine harm is incorrect and misleading, since adverse event reports alone aren’t proof of causality, and the authors didn’t conduct additional investigations that were needed to infer causation. And as explained above, the NNTV doesn’t measure vaccine effectiveness and its use as such in the study is incorrect.
In fact, Petousis-Harris called the study’s results “completely meaningless”:
“The data were inappropriately interpreted to represent vaccine reactions (events caused by administration of a vaccine) and […] the methods used to calculate risk/benefit were also inappropriate approaches to address the research objectives. Garbage went in, got statistically abused, and what came out [at] the end was nonsense.”
READ MORE
FactCheck.org also assessed the same claim and concluded that the study “misinterpreted data and was flawed”.
REFERENCES
- 1 – Dagan et al. (2021) BNT162b2 mRNA Covid-19 Vaccine in a Nationwide Mass Vaccination Setting. New England Journal of Medicine.